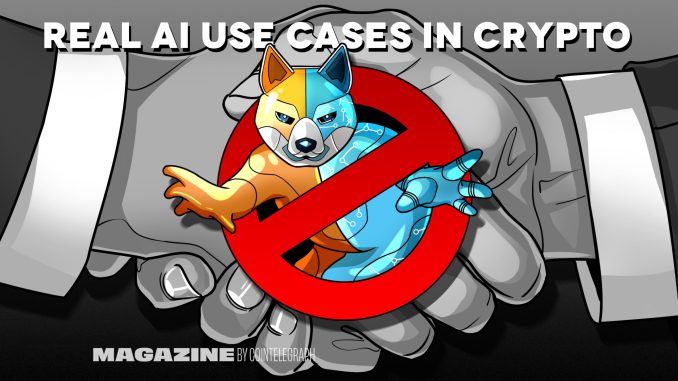
Every day this week we’re highlighting one genuine, no bullsh*t, hype free use case for AI in crypto. Today it’s the potential for using AI for smart contract auditing and cybersecurity, we’re so near and yet so far.
One of the big use cases for AI and crypto in the future is in auditing smart contracts and identifying cybersecurity holes. There’s only one problem — at the moment, GPT-4 sucks at it.
Coinbase tried out ChatGPT’s capabilities for automated token security reviews earlier this year, and in 25% of cases, it wrongly classified high-risk tokens as low-risk.James Edwards, the lead maintainer for cybersecurity investigator Librehash, believes OpenAI isn’t keen on having the bot used for tasks like this.
“I strongly believe that OpenAI has quietly nerfed some of the bot’s capabilities when it comes to smart contracts for the sake of not having folks rely on their bot explicitly to draw up a deployable smart contract,” he says, explaining that OpenAI likely doesn’t want to be held responsible for any vulnerabilities or exploits.
This isn’t to say AI has zero capabilities when it comes to smart contracts. AI Eye spoke with Melbourne digital artist Rhett Mankind back in May. He knew nothing at all about creating smart contracts, but through trial and error and numerous rewrites, was able to get ChatGPT to create a memecoin called Turbo that went on to hit a $100 million market cap.
gm ☕️
As someone with zero Solidity proficiency, I had an already efficient smart contract tailored to my own needs by AI.
I dumped @Azuki’s smart contract into GPT-4 and had it ask me relevant questions.
Disclaimer: Professional human audits and devs are still important to… pic.twitter.com/K4UGfFC5dp
— SV (@0xSMV) March 16, 2023
But as CertiK Chief Security Officer Kang Li points out, while you might get something working with ChatGPT’s help, it’s likely to be full of logical code bugs and potential exploits:
“You write something and ChatGPT helps you build it but because of all these design flaws it may fail miserably when attackers start coming.”
So it’s definitely not good enough for solo smart contract auditing, in which a tiny mistake can see a project drained of tens of millions — though Li says it can be “a helpful tool for people doing code analysis.”
Richard Ma from blockchain security firm Quantstamp explains that a major issue at present with its ability to audit smart contracts is that GPT -4’s training data is far too general.
Also read: Real AI use cases in crypto, No. 1 — The best money for AI is crypto
“Because ChatGPT is trained on a lot of servers and there’s very little data about smart contracts, it’s better at hacking servers than smart contracts,” he explains.
So the race is on to train up models with years of data of smart contract exploits and hacks so it can learn to spot them.
Read also
Features
‘Make sure Ethereum wins’ — Steve Newcomb reveals zkSync’s prime directive
Features
Ethereum is eating the world — ‘You only need one internet’
“There are newer models where you can put in your own data, and that’s partly what we’ve been doing,” he says.
“We have a really big internal database of all the different types of exploits. I started a company more than six years ago, and we’ve been tracking all the different types of hacks. And so this data is a valuable thing to be able to train AI.”
Race is on to create AI smart contract auditor
Edwards is working on a similar project and has almost finished building an open-source WizardCoder AI model that incorporates the Mando Project repository of smart contract vulnerabilities. It also uses Microsoft’s CodeBert pretrained programming languages model to help spot problems.
According to Edwards, in testing so far, the AI has been able to “audit contracts with an unprecedented amount of accuracy that far surpasses what one could expect and would receive from GPT-4.”
The bulk of the work has been in creating a custom data set of smart contract exploits that identify the vulnerability down to the lines of code responsible. The next big trick is training the model to spot patterns and similarities.
“Ideally you want the model to be able to piece together connections between functions, variables, context etc, that maybe a human being might not draw when looking across the same data.”
While he concedes it’s not as good as a human auditor just yet, it can already do a strong first pass to speed up the auditor’s work and make it more comprehensive.
“Sort of help in the way LexisNexis helps a lawyer. Except even more effective,” he says.
Don’t believe the hype
Near co-founder Illia Polushkin explains that smart contract exploits are often bizarrely niche edge cases, that one in a billion chance that results in a smart contract behaving in unexpected ways.
But LLMs, which are based on predicting the next word, approach the problem from the opposite direction, Polushkin says.
“The current models are trying to find the most statistically possible outcome, right? And when you think of smart contracts or like protocol engineering, you need to think about all the edge cases,” he explains.
Polushkin says that his competitive programming background means that when Near was focused on AI, the team developed procedures to try to identify these rare occurrences.
“It was more formal search procedures around the output of the code. So I don’t think it’s completely impossible, and there are startups now that are really investing in working with code and the correctness of that,” he says.
But Polushkin doesn’t think AI will be as good as humans at auditing for “the next couple of years. It’s gonna take a little bit longer.”
Also read: Real AI use cases in crypto, No. 2 — AIs can run DAOs
Subscribe
The most engaging reads in blockchain. Delivered once a
week.
Andrew Fenton
Based in Melbourne, Andrew Fenton is a journalist and editor covering cryptocurrency and blockchain. He has worked as a national entertainment writer for News Corp Australia, on SA Weekend as a film journalist, and at The Melbourne Weekly.
Be the first to comment